Parcl Labs Rental Price Feed White Paper
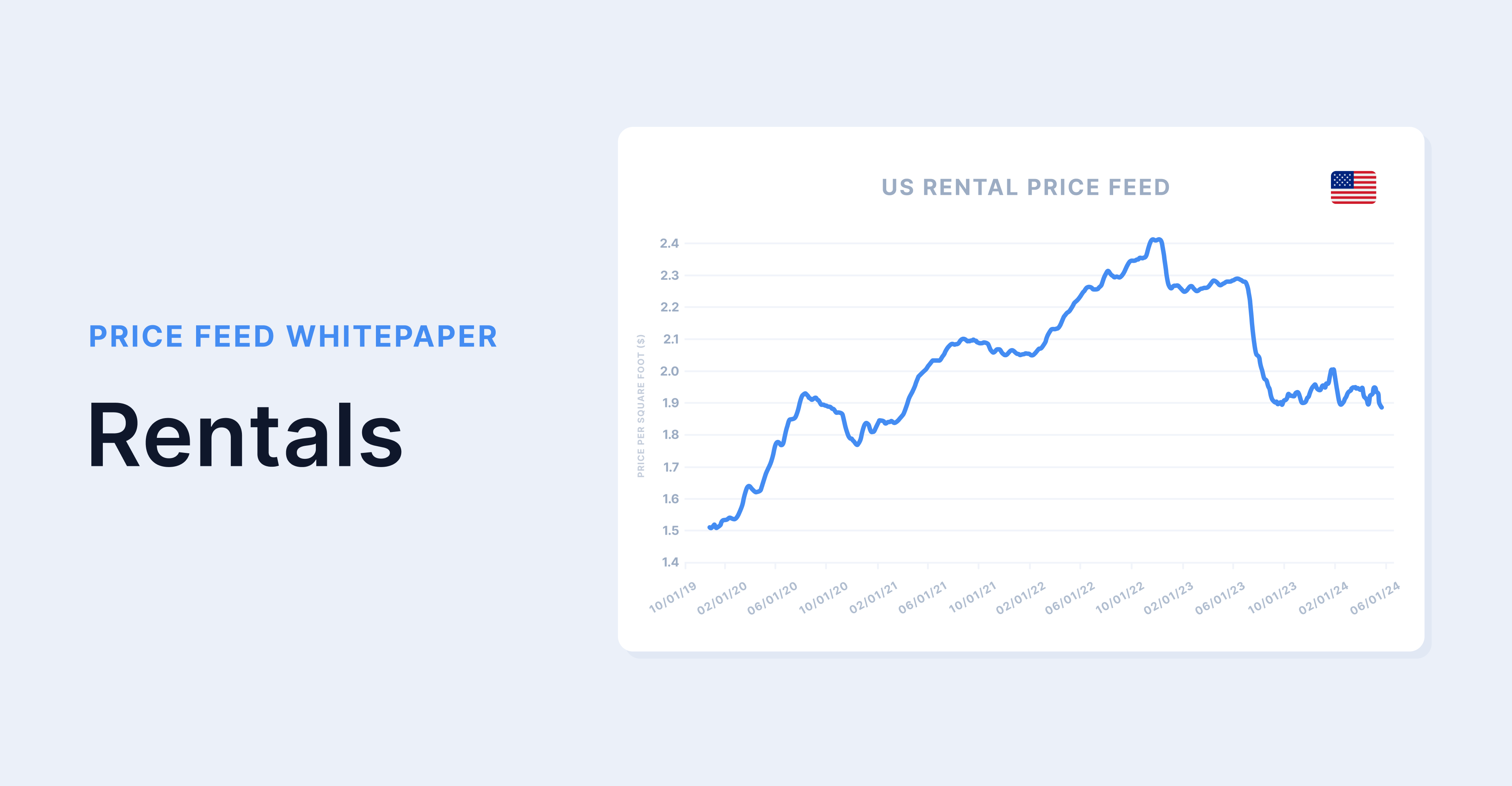
Executive Summary
- The Parcl Labs Rental Price Feed (PLRPF) is an indicator that tracks changes in asking prices for residential rental real estate on a daily basis across multiple markets and property types using a simple metric: monthly price per square foot for rent.
- The PLRPF offers a real-time metric of available residential real estate inventory for rent in any given market, eliminating the need to wait several years for units to be considered in price movements.
- Existing data sources, such as the Rent of Primary Residence component of the Consumer Price Index (CPI), track rental prices with a lag of one month and use sample data that is not available for smaller geographic subsets. Other metrics, like the Zillow Observed Rent Index (ZORI) or the CoreLogic Single-Family Rent Index, both repeat-rent indexes, exclude unseen parts of the market and do not provide a metric to easily compare across markets. Further, the SFRI is published with a 2 month lag.
- Parcl Labs developed an enterprise-level ETL process to ingest, clean, and transform billions of individual data points from multiple different rental listing sources. This process leverages spatial data science to generate price estimates for multiple levels of geography, built on a foundation of 100 terabytes of housing data.
- Our methodology examines representative segments of the market, filters outliers and other irregularities, creates smooth time series, and is tested daily to ensure the quality of our indicator.
- The PLRPF empowers users to make better-informed decisions and can be accessed through our user-friendly API.
The Existing State of Rental Price Indices
The rental market represents more than a third of all household units in the United States. Despite its significance, access to timely and reliable information is often lacking. When information is available, it tends to be outdated, and the underlying methodology limits its utility for understanding current market conditions. Further, studies have suggested that important differences in price movements can arise due to methodological discrepancies, making it hard to pinpoint a single source of truth.
Official government sources shelter component of the Consumer Price Index (CPI), uses a relatively small sample of 40,000 urban residences and only measures price changes every six months. The Rent of Primary Residence index, part of the shelter component of the CPI, is the metric responsible for measuring price movements for occupants renting a unit. The index tracks price changes using a fixed sample of rental units and assumes that the price changes experienced during those intervals are linear, thus ignoring varying conditions on the ground such as the availability of units for new tenants. The semi-annual measurement of price movements is one reason why price changes in housing, especially rentals, often reflect lagged price dynamics, thereby limiting its usefulness in assessing current market conditions. Additionally, to calculate one part of the shelter component , the Owners’ Equivalent Rent (OER), the BLS estimates the value a homeowner would pay if they were renting their unit using comps, a process that is problematic for difficult-to-measure units such as single-family homes. As a result of how the index is constructed more than two thirds of the price movement in the shelter component come from the imputed estimates of rent for owners.
To gauge the impact of the lagged data and the small sample size on the Rent of Primary Residence, we benchmarked our National PLRPF against the Rent of Primary Residence in the U.S. We used the median price point of our National PLRPF on a monthly basis and conducted a correlation analysis. We found an overall correlation of 0.5 from January 2020 to April 2024. However, the correlation coefficient increased when using lag terms of 3 and 6 months, highlighting the lagged nature of this index. Other researchers have found similar results when benchmarking against the CPI.
Figure 1. Correlation of Parcl Labs Rental Price Feed for the USA with CPI Rent of Primary Residence in U.S. City Average
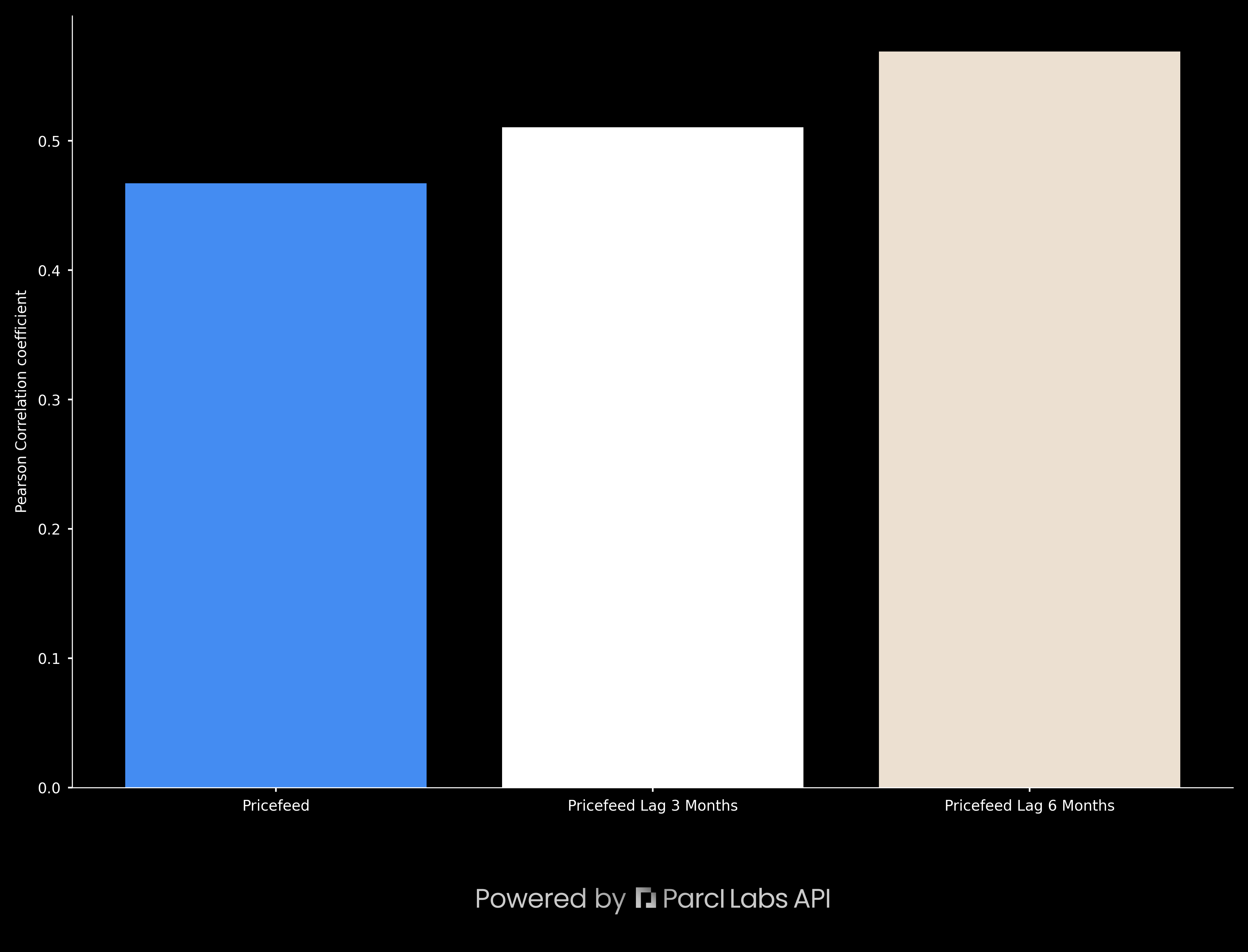
Private indices, like the Zillow Observed Rent Index (ZORI) capture only units that have been observed over time (repeated rentals) thereby discarding actual units available in the market simply because they do not have multiple entries in their sample. Controlling the heterogeneity of the market can paint a distorted picture by ignoring parts of the market that recently became available or that were not listed originally on their platform. We include all properties, regardless of their historical trajectory, and use multiple data sources to build a more complete picture of the residential real estate market.
The ZORI also uses a weighting schema based on how many times a property appears on their platform and adjusts based on how representative that unit type should be according to census data. This information is updated, and to limit price movements, a proprietary smoothing algorithm is used. While these adjustments may make prices smoother, the end result is an index that omits the available supply of real estate simply by virtue of not being represented in their sample space, either due to not being on their platform or by being new construction or lacking rental history. This can be problematic in times of high volatility such as in the aftermath of the COVID-19 pandemic where new units for rental are hitting the market in record numbers.
Another private source, the CoreLogic Single Family Rent Index (SFRI), is also a repeated sales index that looks at single family units (detached, semi-detached, and condos) and creates pairs of rentals to control for the quality of the housing stock. Their data is sourced from a subset of MLS’s in the country, and as a result, they only calculate indices for metropolitan areas where they have enough repeated sales. After the SFRI is calculated for multiple metros a National price index is derived from a weighted average of metropolitan areas. While the index is updated monthly, the underlying numbers are not publicly available, with the price changes being the only data points offered, making it hard to compare with other sources. Even if the numbers were published, unless a user has access to the underlying data to build their estimates back to dollars, this metric is hard to interpret for the average consumer.
Relying on indices that only consider a sample space of 40,000 homes, with prices measured every six months that magnify lagged price points into the future, or repeated sales methodologies with unintuitive weighting schemes that omit vast swaths of the market should not be the default.
Table 1. Comparison of Residential Rental Price Indices
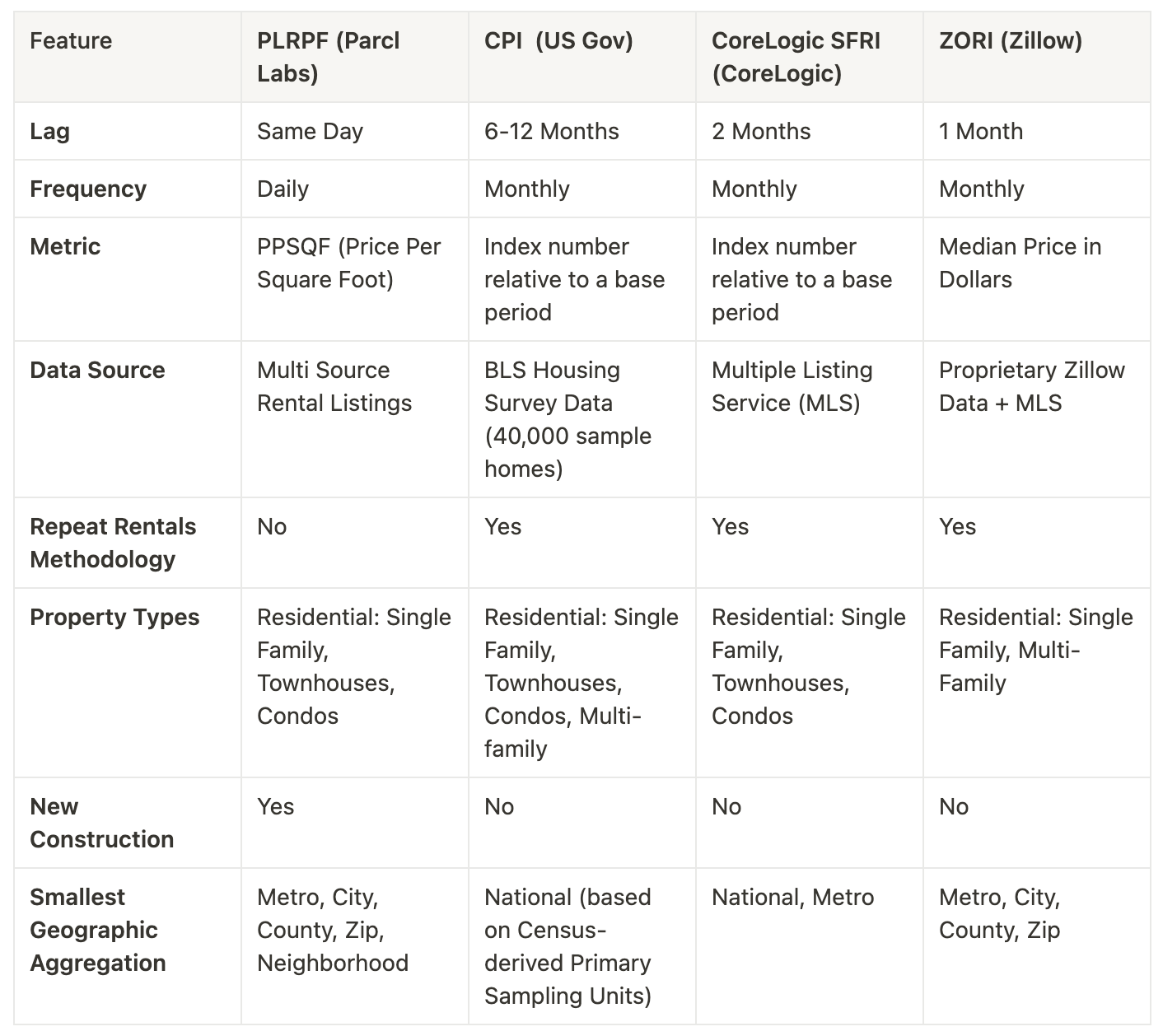
The Parcl Labs Rental Price Feed is Real-Time, Representative, and Intuitive
Today, we are proud to announce that Parcl Labs continues its mission of providing high-quality information to our customers and users with the introduction of the Parcl Labs Rental Price Feeds for several markets across the USA at different levels of geography. This is the first-of-its-kind metric to track a standardized real-time rental price metric.
The Parcl Labs Rental Price Feed (PLRPF) represents the only currently available daily estimate of residential real estate monthly rental asking price per square foot across multiple markets with varying geographic scopes (states, metros, cities, etc.), that examines all available records in a systematic and standardized way. The PLRPF allows users to monitor real-time price fluctuations in their respective markets with minimal lag and with an indicator (median price per square foot) that is easy to understand and can be more representatively compared with other markets. The index provides a real-time picture of available residential real estate for rent, depicting a more complete image of the prevalent market conditions that potential tenants face. An index that reacts to rapidly changing and volatile conditions is more informative than alternatives that only measure small segments of the market.
This approach represents a significant departure from existing solutions, such as the Rent of Primary Residence in the Consumer Price Index, which only provides users with an index comparing the change in shelter value for a given month, the index changes derived from CoreLogic’s SFRI, or the median rental price as seen in Zillow Observed Rents. The PLRPF addresses these limitations by offering a normalized metric (median monthly price per square foot) that can be compared across markets and used to gauge price fluctuations. A square foot of property is the same dimension across all imperial system markets, and future markets accustomed to metric systems will use a square meter as the unit of analysis.
The PLRPF tracks price changes on a daily basis across a variety of markets for all residential property types, allowing users to understand the real-time dynamics of pricing in their markets and in other markets across the country. The Parcl Labs Rental Price Feed addresses the following:
- Provides an intuitive and easy-to-understand metric for tracking the evolution of asking rental prices of residential real estate.
- Generates estimates based on all available information within a given market, rather than relying solely on a subset of transactions and with methodologies that are hard to replicate.
- Standardizes and integrates data from different recording systems to create a single source of truth.
- Reduces asymmetries in access to residential real estate data, thereby empowering users.
- Offers an index that adjusts to the velocity and volume of transactions occurring within any given market to provide an unbiased assessment of real estate prices.
All of this is possible thanks to our state of the art data warehouse where every data point is subjected to a rigorous process before being used in the calculation of the PLRPF.
Data Collection
Like any model, the first step in creating our price indicator for real estate starts with high-quality, clean, and standardized data. The process begins with collecting data from a variety of rental data sources. Our data universe encompasses information from new construction, repeated rentals, and properties without previous rental records. We include all residential housing types, such as single-family homes, townhomes, and condos, offering a more accurate depiction of real estate markets. We do not limit ourselves to a single source of data as we collect from multiple listings sites as well as private sources.
Unlike the CPI, we collect billions of datapoints across all geographies from a variety of sources thus guaranteeing a more complete and real-time snapshot of the residential rental real estate market. This allows us to look at millions of data points when generating a price estimate for a given market and only selecting the observations that pass our ETL process. To calculate a price estimate for a single day for our National PLRPF day we look at half a million points.
Figure 2. Data Points Available for PLRPF Calculation on a Given Day
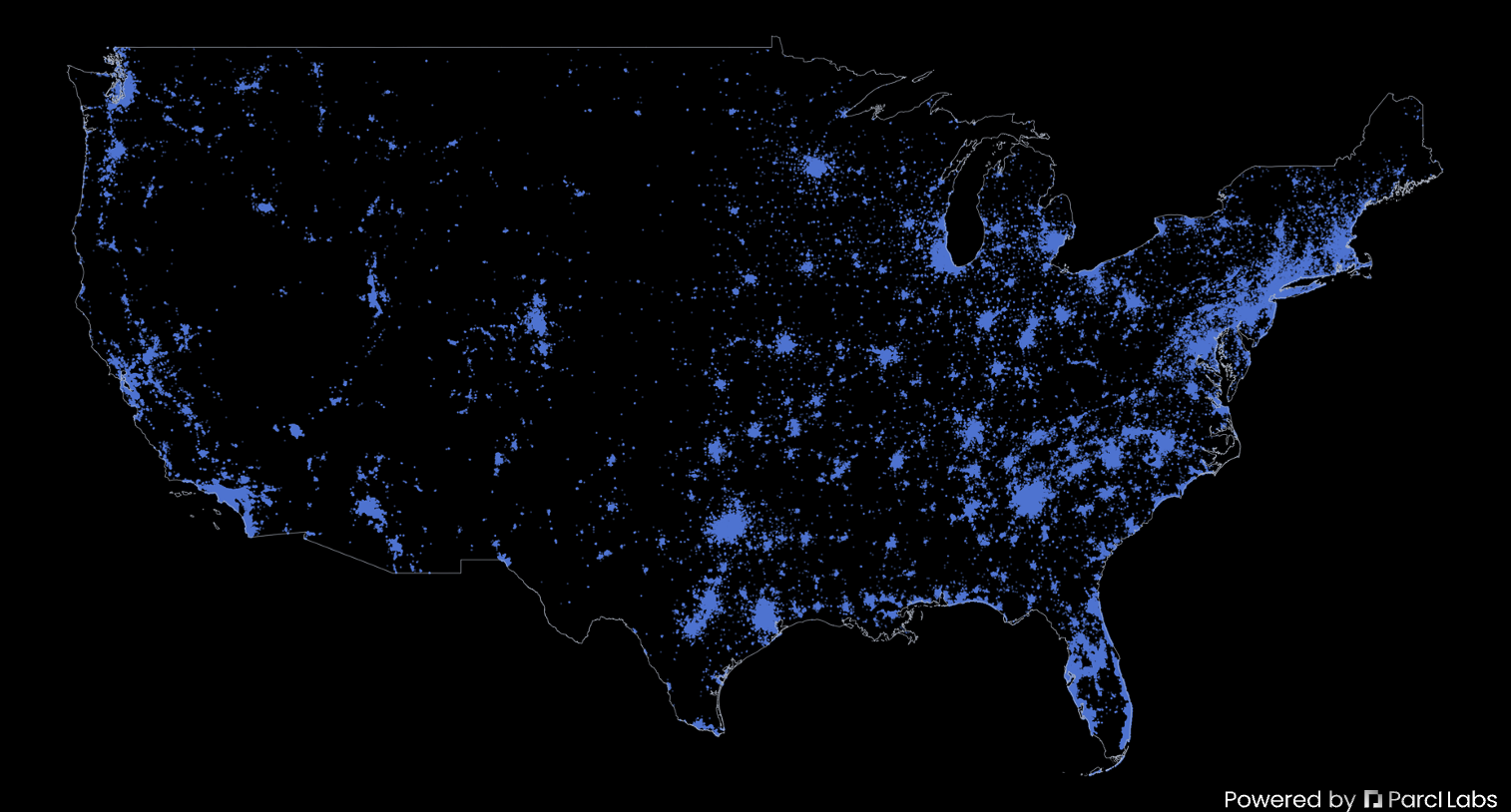
Data Integration, Standardization, and Processing
Just as we do for our Parcl Labs Price Feed our data is processed through our ETL process described here. Once we have ingested the data points, it undergoes a validation, integration, standardization, and enrichment procedure:
- Cleaning, Deduplicating, and Standardizing: We clean, deduplicate, and standardize information to ensure the data for each rental unit is up-to-date and accurate. This step is crucial when collecting information from multiple data sources as it eliminates redundant information.
- Address Validation: We validate the accuracy of addresses, particularly for historical rentals, asserting it’s a valid US mailing address, to prevent duplicate data entries and ensure data integrity.
- Data Reconciliation for Historical Records: We reconcile data to ensure consistency over time for each property record, addressing the unique idiosyncrasies and time lags of each data source.
- Geospatial Mapping: We leverage geographic information systems technology to classify properties into various market types using government provided legal boundary definitions. This allows us to assign properties to multiple levels of geography, ensuring our price feeds are scalable and accurate.
Once the data standardization process is completed we have a unique state-of-the-art database to build scalable and timely PLRPF for any desired level of geography, a necessary step before we can build the most timely residential real estate rental data.
Parcl Labs Rental Price Feed (PLRPF) Methodology
The Parcl Labs Rental Price Feed (PLRPF) follows a multi-stage approach to deliver reliable and consistent price estimates. We take the data prepared in the previous step and transform it into a final time series for each market. For the PLRPF we include observations that fall within a price range and surface area that reflects the median market and includes property types such as single-family houses, semi-detached units, townhomes, and condos. Our data reflects available units and current market conditions, capturing the rapidly changing dynamics of rental markets, particularly in constrained markets.
To arrive to our price estimates, we follow a process that involves two main stages: correcting for volatility and market idiosyncrasies, and combining multiple data sources from our warehouse. We rigorously test the estimates produced to confirm the reliability of the data.
Time Series Correction and Smoothing
Our correction method is designed to be robust against outliers, adjusting our daily estimates as detailed in our Price Feed White Paper. We restrict observations to the 25th to 65th percentile range to minimize outlier effects. To arrive to these numbers we conducted thousands of experiments to determine the optimal parameters for the PLRPF, balancing data richness with control over excessive volatility from outliers.
Figure 3. PLRPF Experiments to Find Optimal Time Series for Washington DC

The process of removing outliers to guarantee reliable data points also needs to account for data sparsity. As part of the experiments, we also tested how many days a backpropagation window should have to ensure a sufficient level of information for any given market. For each market we ran hundreds of different configurations to find a tailored window based on transaction volatility. The following figure illustrates the differences in transaction volume between Austin City and the Austin MSA .
Figure 4. Number of Observations Available to Calculate the PLRPF in Austin City and Austin MSA
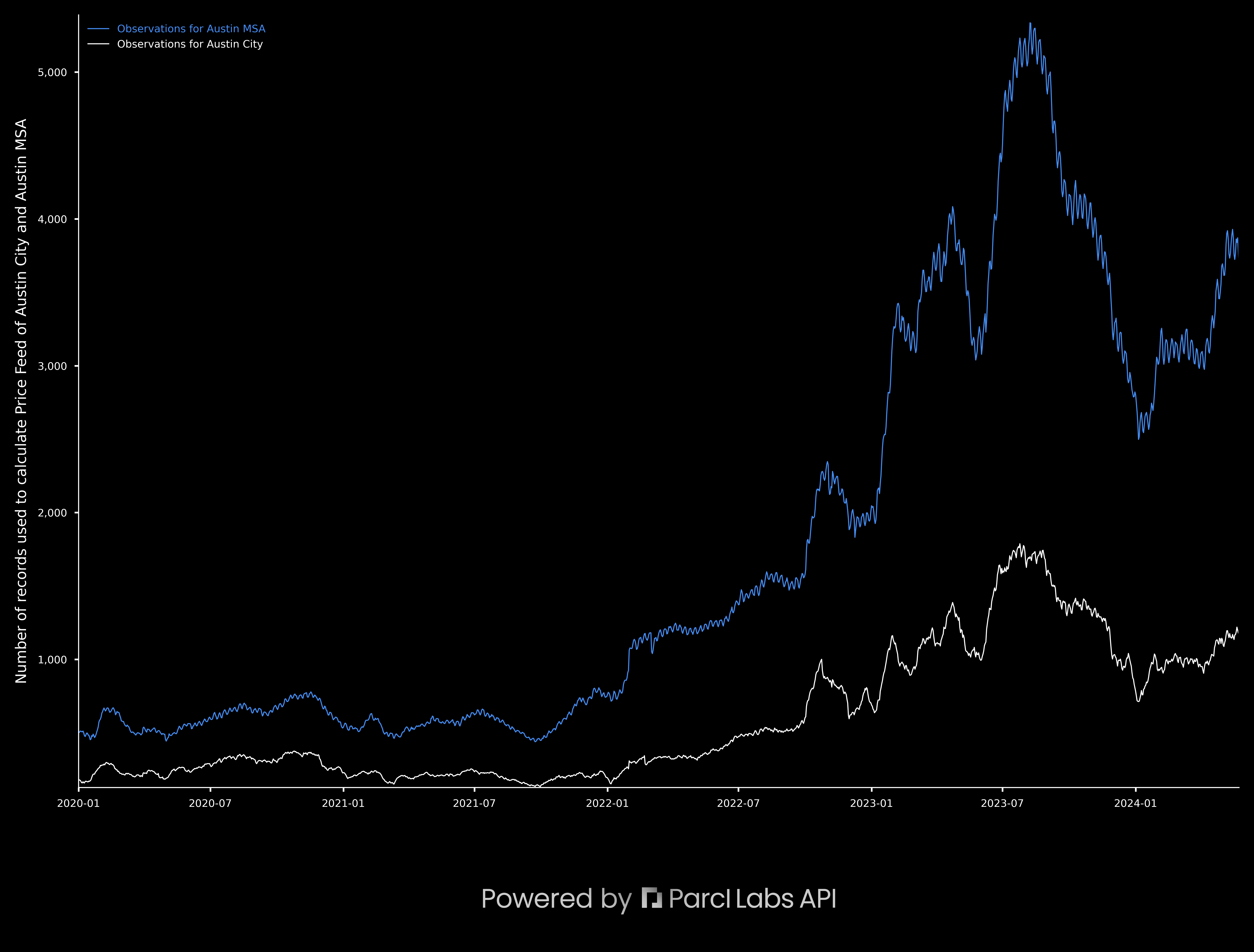
After that sample space has been built we calculate daily snapshots of median asking rental price per square foot for each market, using the appropriate window for each market. This process can be represented by the following formula:

where ti is the window for market i.
By using a moving median, we are able to smooth out price fluctuations, capture short and long term trends, and regularize market idiosyncrasies. Finally, we employ a 14-day smoothing process to further minimize the impact of market fluctuations.
While seasonal adjustments are common for monthly and quarterly series, there is no consensus on adjusting daily series due to significant intra-week and market-specific variations. Therefore, developing a one-size-fits-all approach is challenging.
Dynamic Testing for Price Irregularities
We continuously test the consistency and reliability of our data for each market available in our API before updates. This testing accounts for abnormal behaviors from various data sources, local market idiosyncrasies, and geographic factors that may influence the volatility of our series. Our tests look for:
- Substantial changes in the volume of transactions in the short and medium term.
- Changes in the distribution of prices in the underlying sources that deviate from historical patterns
- Test for short term fluctuations in our estimates to avoid a volatile estimate.
This process results in clean inputs and model outputs that are tested daily to guarantee accurate daily estimates. By continuously validating and refining our data, we ensure that our rental price feeds reflect the most current market conditions. This rigorous approach provides users with reliable, real-time insights into rental price trends, helping them make informed decisions.
Conclusion
The Parcl Labs Rental Price Feed (PLRPF) revolutionizes access to residential rental real estate data. By meticulously tracking daily price changes in residential properties across the USA, the PLRPF provides users with timely and useful information to inform rental real estate decisions.
We offer the only price feed that tracks existing market conditions on a timely manner, for a multitude of regions and geographies across the USA and for all types of properties. As a result we offer unparalleled information on a volatile market. Our metric is easy to compare across regions and can be used in conjunction with other economic indicators.
At Parcl Labs we continue to work on products to accurately reflect the real estate market. And while this index does not currently consider large multifamily apartments given the volatility in their availability and pricing, we are working to bring this index online.
With our user-friendly API, the Parcl Labs Rental Price Feed empowers users to make confident, informed real estate decisions. Sign up today and unlock the potential of advanced real estate analytics.